Improving Toolpaths with Artificial Intelligence
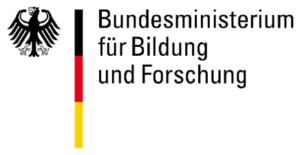
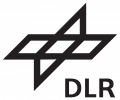
The project “KI4Toolpath” is funded by the Federal Ministry for Education and Research (BMBF) and the and the German Aerospace Center (DLR) under the project no. 01lS22021B.
Details
One-off and small-batch productions are a challenge to many additive manufacturing processes, but they also provide the needed flexibility for production. Machine Learning (ML) opens up many possibilities to improve manufacturing in this context. The aim of the project KI4Toolpath is to combine process-based sensor and geometry data with a specialized ML solution for the adaptive generation of toolpaths. The expected results of the project include less geometric deviation of products, the reduction of defects in one-off productions, increased contour accuracy and shorter lead times.
Industrial production is subject to continuous change towards one-off and small-batch productions, shorter production cycles and a previously unseen product variety. This change is taking place while equal or improved product quality is expected. These circumstances require companies to provide flexible production environments that provide reliable technologies that deliver results in a predictable time and quality for variable workpiece geometries. The current industrial practice already demands experienced machine tool operators with a deep understanding of the process, and with increased expectations, processes become more complex, thus requiring even more knowledge.
While the demands on the industry and operators are rising, both experience innovation through data-based methods for the prediction of results, toolpaths and controlling. Especially machine learning (ML) opens up almost endless possibilities to reduce the consumption of energy, time and material resources.
Within the context of one-off and small-batch productions, machine learning methods are yet to be integrated. The main reason is the poor access to data for geometries that are individually manufactured in small quantities. This might be overcome by researching the potential of machine learning since the approach already provided excellent results under similar conditions.
Two approaches are going to be used to employ machine learning during additive processes. Firstly, artificial intelligence (AI) algorithms are researched to generate the adaptivity of toolpaths to events that occur during manufacturing processes. Within the first approach, AI processes such as Long Short-Term Memory (LSTM) and Convolutional Neural Nets (CNN) are used. This is secondly complemented by the integration of geometry information of the workpieces through data compression. The data is subsequently made available to the AI.
The methods of the project are applied to a specific use case, wire arc additive manufacturing (WAAM). The process has the advantage of enabling fast, flexible, and material-efficient one-off or small batch productions of big and geometrically complex semi-finished products with high deposition rates of at least 5 kg up to 10 kg per hour.
While production might be greatly improved and advantages may be reached, the use case also poses some challenges to the project. One challenge is staying within the needed shape and dimensional accuracy which typically fluctuates due to the thermic inhomogeneity of material application. This is normally compensated by process experts who manually adjust the calculation as well as the process parameters and strategies of the CAD/CAM software. The ecological and economic efficiency of WAAM needs to be increased in order to really deploy all advantages of the technology.
With the developments, several measurable advantages are expected. Among the advantages are reductions of geometric deviations by 20% as well as reductions of defects by 30% in the case of one-off production. Additionally, higher contour accuracy will allow reducing the application of excess material by 50%, and faster processes that eliminate latencies will decrease the lead times of semi-finished products by at least 20%.
ModuleWorks is largely involved in the research of toolpath strategies and their combination with artificial intelligence.